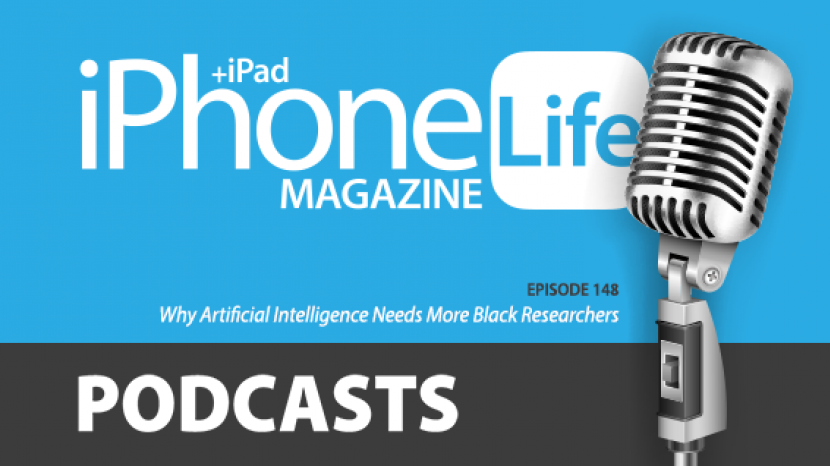
In episode 148 of the iPhone Life Podcast, Donna interviews Krystal Maughan and Hassan Kane of Black in AI, a nonprofit that mentors Black researchers in the field of Artificial Intelligence. Learn about the exciting field of AI and why creating inclusive AI algorithms and workplaces is so important.
Click here to listen and subscribe. If you like what you hear, be sure to leave a review. And remember to tune in every other week to hear our editors share with you the latest Apple news, best apps, iPhone tricks, and coolest accessories.
Related: Episode 147 of the iPhone Life Podcast: Apple Releases New Macs with M1 Chips
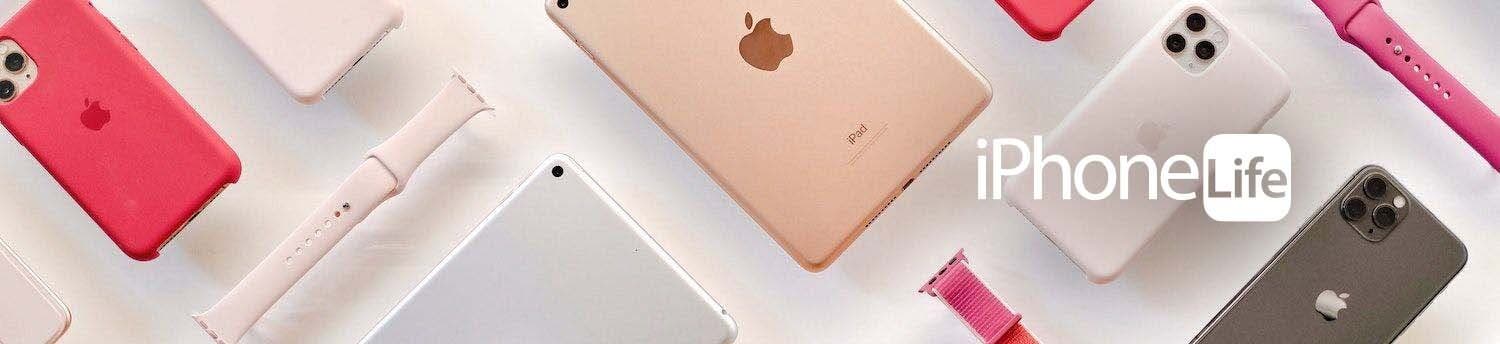
This episode was brought to you by Informant 5. Let's face it; Apple's Calendar app is basically a to-do list. You need an app with the power to tackle your busy day. Informant 5 is the best calendar app and task manager you’ll find to help you manage both your work and personal life. Manage projects with tasks and notes, sync your calendar among all your devices, get a user-friendly 30-day calendar view, and much more. Get Informant 5 for your iPhone and iPad today.
Question of the week:
In what ways do you use artificial intelligence on your iPhone and in your life? Email podcast@iphonelife.com and let us know.
Useful links:
- The iOS 14 Guide: Master Apple's New Widgets, Tools & Features
- Join the iPhone Life Facebook Group
- Insider walk-through: get a sneak peek of members-only benefits
- Get the Insider discount for Podcast Listeners
- Sign up for the free Tip of the Day Newsletter
- Email the Podcast
- Subscribe to iPhone Life magazine
Transcript of episode 148:
- Hi and welcome to the iPhone Life Podcast, I'm Donna Cleveland, editor in chief at iPhone Life.
- And I'm David Averbach, CEO and publisher.
- This week, we're doing things a little differently, we have a special guest for you, and I will cut to that interview in just a moment, but first we wanted to tell you who our sponsor is for this episode.
- So today's sponsor is Fanatic and they have an app called Informant 5, and if you haven't heard of it, I really recommend you go check it out. We like to say it's the calendar app that Apple should have made. And they just do a lot of things in the calendar app that make it really easy to use, in a way that I personally just found Apple's built in calendar app really tricky to use. One of the main differences is they can actually combine reminders and a calendar functionality into one app, because so many times that line is really blurred of what is a reminder and what is something you're scheduling. If you're like, remind me tomorrow that I have a vet appointment, is that a reminder on your phone or is it a calendar appointment? So that's one of those things that they combine it and they have it in a really user-friendly way. Also their app works not only on iPhone, iPad and Mac, but also on PC. So you can be cross-platform and Android if you happen to have an Android. It's free to use, they do have a premium subscription, but make sure you go check it out on the app store. It's called Informant 5 or we'll link to in the show notes at iphonelife.com/podcast.
- Thanks David, and now for our interview.
- Enjoy.
- Today we have special guests from Black in AI, a nonprofit that is promoting collaboration and increasing diverse voices in the field of artificial intelligence, especially promoting having more black members in this space. So today we have with us Crystal Morin, and she's a PhD student at the University of Vermont, and is researching provable fairness, differential privacy, and machine learning. And at Black in AI, she's helping to organize events and mentoring people in this space. So welcome Crystal.
- Thank you. It's nice to be here.
- And we also have Hassan Kane and he's the lead data scientist at Entropy labs, that one's a little hard to pronounce. He directs machine learning efforts to improve customer support interactions there, and at Black in AI, he is the community programs lead, welcome Hassan.
- Thank you , great to be here.
- Just to get us started, could you tell me a little bit about how you ended up working at Black in AI?
- So my journey started when I joined grad school in 2019. So I had moved across the country and I felt fairly isolated in my first semester, and I happened to get a travel grant from NeurIPS, which is the largest AI conference in the world. And through NeurIPS, I was able to meet several individuals on Black in AI because everyone was telling me I had to go to Black in AI's formal events. And when I attended Black in AI's formal event at NeurIPS, it's a workshop with research and full of community and support, I loved it so much that I thought that I had to, it was really important to me, in terms of providing support, and in helping me see that there are other people like myself who were professors and on the other side of the PhD, and so I made a deliberate effort to try to help and support other persons who are like myself in graduate school. And I became involved in Black in AI that way.
- Yeah. And for me, my involvement in Black in AI go back to the first workshop in 2017. So at that time I had a paper that I presented at another workshop at NeurIPS in 2017, and found out about Black in AI and Jimmy Gabriel reached out and say that I was also able to present my work there, and when I saw the wonderful community and its people, it really made a strong impression because, at that first conference, they indeed took care of registration fees, and created even a dinner and a community for all the black researchers from all over the world, and in college, I did a lot of community building, and so I was looking for something like that in my professional career, so it was really helpful to find such an effort, because I knew from early on the power of community building. So I started helping out with many things like admission, social media, and now I help with basically a lot of the programs itself that the community wants to initiate. And so that's been wonderful to be in service of such a community for almost three years now.
- And I was wondering if either of you could jump in with an answer for this, if you could just summarize for our listeners, what is the mission of Black in AI and why it was founded?
- So Black in AI in terms of its general mission, is this community or this forum for sharing ideas, fostering collaborations, and discussing initiatives, to increase the presence of black people in the field of intelligence.
- Our podcast listeners are all Apple enthusiasts. They all use Apple devices and are already interacting with artificial intelligence in ways they may or may not realize. I was wondering if you could walk us through a little bit, like what is artificial intelligence? For people who don't know, and how is it already benefiting our listeners' lives potentially?
- So artificial intelligence is a very data-centric process. So it's... we call it intelligence because it's a form of learning. So instead of explicitly programming a system or a computer system, you're taking massive amounts of data and having the computer make a program that learns from the data and produces a prediction or does a specific task. And the way it learns is that every time you do an iteration of learning, it corrects the correct prediction, or the intended prediction, from the margin of error or how far it missed the correct answer, and so it does this over and over until it succeeds in predicting or performing the task with a small of a margin of error as it can, and because of that, we turn that intelligence because that's similar to how we might think about intelligence and how the brain works, and that's kind of why it's called artificial intelligence.
- And some of the use case you may see already in your phone is that, if you're using Siri, that performance speech recognition, that's a form of artificial intelligence, if you look at your photo and you recognize that it can sort them based on the people who are in the photo, that's a form of artificial intelligence too. Machine translation is also a form of artificial intelligence, because there is not exact rules to translate from one language to another. So if you want to, you cannot write exact rules to translate, all you can do is show a computer, a lot of example of translation among pairs of language, and hopefully it will pick up the patterns. Another area too, is if you use a lot of applications such as YouTube or Amazon, if you buy an item, you will see that you are often recommended content that's more or less relevant, because it learns from your choice and whether or not you click on the video which are recommended, or the items which are recommended will further tailor, it will tailor it to your choice. So those are already four example, of how artificial intelligence is already powering a lot of user experience.
- So is it artificial intelligence that makes it, I feel like these days, I'll have a conversation with a friend and then somehow ads are being like tailored to me, about a convo, I swear it happens sometimes even when I'm not searching things. Do you know anything about that?
- It's funny actually, there are a lot of anecdotes around that, a lot of the companies I've always said that they're not really doing any of that, but it's true that like, even for me, it often happens, and it cannot even be like a coincidence by voice, that you don't like, the device is there, and then somehow two or three days later, you will start popping up. And clear, the official statements from the company is that they're not actively monitoring this conversation, but yeah.
- I know. I wonder sometimes, but yeah. So that's really interesting to let people know that things like facial recognition, all of that, already is artificial intelligence on our phones, but I know there's a lot of more like futuristic, forward-looking ways that AI, like there's a lot of promise in this field. Could you walk through some of the ways, kind of cool things that AI could be bringing our way in the future?
- So one of the things, and particularly from a person that I went to, I knew in my first semester. She's at UCLA now, but she's working on using AI for gates or walking, so that the AI can be used for prediction in terms of predicting when someone who's elderly, for example, is about to experience a fall and correcting for that, so I'm very intrigued by the humanistic aspects of AI, because I think that there's a lot of potential for AI to be used to help humans. And it's not just, I know there's kind of a generalization that they're just used to make ads and make profit, but there are very collaborative and participatory applications of AI.
- And two more applications, or I guess, two or three more applications, which are exciting and in the work, there is also autonomous vehicles. It's actually a topic that I worked on at Uber advanced technology group, where the promise there is in car or planes or boats that drive themselves. And what's often interesting that a lot of the autonomous vehicle conversation is focused on the car aspect, but even for boats, it's applicable, as well as planes. And actually most of the planes already are like, not exactly driven by the pilots, so that's like one aspect. Another one is around NLP, and for example synthesizing scientific literature to make evidence-based recommendations. So the dream for many researcher is, the rate at which we produce research and knowledge, almost no one can keep up with it, and so if you can have system which are able to read paper, summarize papers and recommend given a certain intention of a user, that would really help to synthesize and personalize knowledge, and a third one is around the AI more in the physical world, so for scientific experiments. There are many items or say chemical reactions or material with some property that we want to design, but the typical process is you design something, you make a computation then you iterate, but often there's that full loop of designing materials to be completely automated, where in a lab setting, the properties can be tested, and then there is a generative model that say, I want a material that is very light and is a very good conductor, or I want a material that's a super conductor, all of those things, and so really blending physics knowledge with experiments to really help design material for better battery or things like that. That's also another coming use case that people are working on several pieces of.
- Cool. The one of synthesizing information, it sounds like my job as an editor could be threatened in the future by AI, huh?
- Well, augmented and--
- Already. No, it sounds pretty amazing. I was wondering if you could talk a little bit about what the current landscape is in the field of AI when it comes to black researchers and other racial groups, how are they represented in this group? I assume that the need for a nonprofit like Black in AI, would show that there's a lack of representation.
- So there is a lack of representation throughout. So not just in fields like technology or in research, but specifically in terms of positions of power in these companies that make AI, and in terms of representation of the general population, I believe it's 13% of persons are black in terms of the elements or the sampling of a population, but this is not the case in certainly the black research space or the research space in general, and we can do a lot better. The other issue is that there is a dearth of black faculty. There is an, and this is specifically true in tenure track positions. So what you find is that you have a high percentage of adjunct faculty who are black, so they don't have benefits and they don't have the same kind of salary as the tenure track faculty, and this is a huge problem because a lot of graduate students, particularly who look like myself, they experience something called identity threat. So they come in with imposter syndrome and graduate school is hard, and one of the ways to mitigate or mediate identity threat, and to make them feel like they belong and can make it through the process and support them is by, research has found is by having black faculty at universities. So if there's a lack of representation, then that kind of perpetuates this pipeline, whereby black researchers or people who want to get into the field to create a more inclusive design process and be involved in AI research, are kind of stymied because they don't have that support.
- Yeah. And if you want to further break down the trends is that, I mean, to start off, to start off with what Crystal mentioned, overall under-representation in many aspects, and the trend is that for example, the community grew from around, you know, few dozens of people, to now 1800 people identifying as black, who are either taking more than two machine learning class, or work on substantial project and are now entering the field, and the Black in AI community provides then a space for these people to relate to one another, and socialize, and feel like there's a place for them to ship the research question. And if we also take another look at some of the researchers on the African continent, some of the obstacle have historically been, for example, the ability to participate in international conference, travel. So there were like, a few years ago, there was a big scandal, actually two years in a row, there was big scandals where, one of the big thing that Black in AI has done actually, has been for say, researcher who are based abroad, actually Black in AI, if you get a paper at this conference, Black in AI will actually pay for your ticket and the hotel registration fees, which is a huge, you can imagine this is a huge deal, if you are researchers in a developing country now being able to network with like-minded people in the field and be able to actually learn what are the latest ideas, the latest conversation, and so Black in AI actually has taking steps to pay for the hotel and ticket for those ones to attend those conference, but despite that, there were actually something like, I believe a third of the VISA were rejected actually. I'll say for those were coming from say, Nigeria, Tanzania, South Africa, Trinidad, Brazil, like they were actually VISA that were rejected, even though the people had their papers accepted at the conference And so that has been like one example of obstacle because, of course, like in the research community, like it's really important to be attuned to what is the latest conversation, because that's what is present in the latest conversation that would drive the research agenda for the next year or two, and so even if you have the ability, if you're not aware of the latest conversation, you don't go into these in-person meetings, the relevance of the work can be hard. So like Black in AI has really helped to tackle some of those obstacle, and the numbers are improving now, I would say from like a couple of dozen people, to like 1800. Another example, actually, there's a platform called Zindi Africa, that basically they do a data science platform, a data science competition platform specifically targeted towards research on the African continent, and they have reached 20,000 users in over like a year, like they were founded in May, 2018, and so they have like reached like 20,000 users over a year and half, because people are really seeing that the opportunities are there and the problems are relevant to them, and so they are really tapping into their time to self teach themselves a lot. As a person, like Crystal and I work on admission, so we really see this amount of talent that's often self-taught, or, you know, I'll stick in a lot of Coursera classes online, and they are looking for other people, that may not be in their city to relate to, and so we have so many stories like that. We can literally spend the entire afternoon talking about someone that may have studied mechanical engineering at some point, they work a job, then they take five, six Coursera classes, and then what's next, right? If you are someone in a community where there's not much activity happening, that could be a barrier, but now that there is a community like Black in AI, they can actually join the forum, introduce themselves, initiate collaboration, and that has been really powerful in empowering that acceleration of the presence.
- That's very cool. Yeah. You mentioned somewhere in there, something about a group of 1800 researchers, is that the membership number for Black in AI or?
- So, yeah, we're around, the membership number is around that, but actually that just include the black people. We also have allies on our forum, and so there are, like allies identify as say, faculty members at other university, young professional want to help, and that number, when you add allies around that, like 2,700 plus. Yeah.
- And Crystal you'd mentioned before too, 13% being the general US population of black people, but that the numbers in AI were a lot lower. Do you know what they are or happen to know what they are? That's okay.
- Well, yeah, the only thing I remember is the statistic that Reditz made, which was that in 2019, when she received her PhD or was it 2018, she was one of, so only 11 persons received, black persons, received their PhDs in the US, and only four of them were female. And she knew all four of the women who had received their PhDs in the US and identified as black. So, I mean, it's really pitiful, the numbers are, we can do a lot better, and part of that, as Hassan was talking about, it has to do with mentorship, and I think that Black in AI is a great space to connect persons together so that we can have better opportunities for mentorship, which leads to success and increasing or having more representation within faculty and an industry of researchers working in AI.
- Right. Yeah. And, you know, so far I'm hearing a lot of just in terms of fairness and equal opportunity and feeling like this is something I can do too, this mentorship is so important, but then there's also the whole other side of it that artificial intelligence can have bias within it, and can be harmful in that way, if there's lack of representation. Could you talk about that? What's some of the harm in that?
- When the people who are in the room and decide the project have a huge influence on the project outcomes, because that includes the awareness of the assumption that power, the data that has been gathered, the use case and its impact on different communities. Because if people often go with the mindset of move fast, break things, and we'll iterate over time, it doesn't always lead to thoughtful development as far as what are the implications, what are the real life implication of break things or things that break when they become elements of critical decision-making? And one of the most prominent example that has been shown by members of the community, including Timnit, Joy, and Debs, and many other who work in fairness has been that for example, facial recognition algorithm actually are not as good at recognizing both the gender and often even the person, based on the skin color, and that's often due to how the data sets which are gathered and the way the applications are designed to be tested. But more importantly, I think they were like lots of other examples that show that they were able to match pictures of like Congressmen, black people from the Congress. 40% of them match with mugshots, which are not the people who are, you know, I mean, just matching them with any random mugshot. So if you imagine a predictive posting use case where someone is now locked out of a store, because his face has been matched with a mugshot, whereas it may not be him or her, like you could see how it can lead to a lot of problems. But that's, those are actually some of the most visible example. They can be other ones, and part of it is that it's in a way that, and so actually another example of that was there was Amazon that made almost like a resume ranking system, and it learned over time to actually like discriminate against women, and part of it is because if you look at the current setup and the current representation, and if you say, learn from that, then you will learn to, you will learn the current setup, versus, maybe you would not want to learn from that, and you will want to say, we want to actually take up, if we want, if we mean wanna be able to consider applicants from different backgrounds, by definition, they'll be underrepresented in your data set. And so they actually immediately suspended it. And so those are example where, in some case maybe want to reinforce the trend, but in some other case, you actually don't want to reinforce the trend. Especially if you say you want to consider applicants, which are not like the one that we have in house, it means that you have to be relatively open-minded, and if you learn from the patterns of the choice that you've made in the past, then you're going to repeat them, literally those who learn from history are condemned to repeat it. And then the third point I want to add actually, is this has been very interesting, this conversation on facial recognition, because it's one of the cases where people may not necessarily wanna be included. Like I think there were like great quotes on Twitter that say, don't confuse equity with like representation, because in that case, it's actually a good thing that these technology may not work, and some folks in the civil rights side, I've actually taken the opportunity to question the wider deployment instead of saying, let's develop, actually, data set that includes better people, I mean, that includes more representation so that everybody now can be face scanned, there's been actually a program say, well, actually we don't want to be face scanned and be included in your data set. So it was also this very interesting conversation that's ongoing.
- I was gonna say, is that kind of speaking to, if there's very accurate data being kept on everybody for like, if the people in power are corrupt, then that could be used against you. Is that the concern? Like, what is the concern around having better facial recognition?
- So, I mean, one of them is the, and often, a lot of the community says that when the technology development happens, technology development doesn't happen at the vacuum, in a vacuum, it happens within an already existing social context, and in the US, the social context is one where there are already historical issues with respect to where policy is deployed and which population it monitors and the tension that it has created. And so when the development of technology happened within such a context, you will further exacerbate the tension, which already existing between the police and some communities of color, and so in that case, a lot of the civil rights people, and wider social scientists, were right to further question whether that's something that we want, because we know that it's, people may want to start deploying it in schools and everywhere in tracking all sorts of behavior, and is that the kind of society that we want? And the fact that in that case, the technology wasn't mature, didn't work, allowed people to have that honest conversation and say, is that the world we want to be headed, or do we want to completely change the paradigm of how we imagine the relationship of police in this community being one of over scrutiny and things like that? And so, I think like it was one of the case where the next step wasn't just about technological improvement, but thinking about how do you wanna change? And we will see that conversation being mirrored nationally, like, how do we really want to change those power dynamic and relationship? Because it's known that those technology will be, have the potential to be abused against certain groups, and that's where the conversation has been happening.
- Crystal, do you have anything to add for that one? That was a great answer.
- Yes. That was a really good answer. I guess you kind of address, so there are several problems with, or several complex layers of complexities within bias, so you have the data collection process in which persons tend to, depending on who's collecting the data, they may have a tendency to categorize things based on their impression of the world, so then codings are an issue. And this is particularly an issue, for example, in police reports that only have genders as male or female, if a person just identify as male or female, and we also have the general modeling process. So based on the data, there's a lack of representation or a sampling bias, and the issue, so in June of this year, June 24th, there was a gentleman who was falsely arrested. I believe that the article was in the New York Times and because of faulty data and using things like gang databases as part of the training, data samples which leads to higher margins of error or grainy surveillance video, because it was just a lack of representation of black persons in the data samples themselves, and so that perpetuates into misclassification when models are made, or algorithms are trained on the data. So there's that, the modeling aspect as well, and so it can lead to incorrect predictions, which penalize already underrepresented or marginalized individuals. So it's an entire pipeline of issues that are compounded that lead to punitive relationships and a lack of trust between technology and algorithms, and the people that they're used against or weaponized on.
- And, yeah, and as far as the power of question goes, it's like often even the recourse to these, I think like often, you know, even for example just in the simple case of a recommendation system, you cannot really question or modify what recommendation system suggest. Those system, they're like a lot of work around what people call participatory approach to machine learning, where users have the room to review the inputs and modify the inputs to these decision systems. And because in that case, because the deployment would be in a way that the person will have a hard time questioning, because say the decision has already been made of say, locking him out of a store or something like that, and so the way that, how do you go and question that, right? How do you go and say, this system that has misidentified me, now, like I cannot do XYZ, how do I go and question that? Who made it? And so those things were also not clear so that's one of the questions around who has power to question and ask the information to be reviewed. So that's also another question.
- Lack of transparency, basically?
- Yeah.
- One thing with that, as well is, I'm wondering from your point of view, like, social scientists and civil rights activists are having concerns about collecting this data, but it does seem like on the other hand, having it be more accurate, would have a lot of positive effects as well. Do either of you have an opinion that you would weigh in on what you think the right course of action is forward? Or you could pass on that if you would rather not state an opinion.
- So, I mean, I can start. I mean, it's all use technology. I mean, in an ideal world, like you want a way for people to be able to decide what are the, because what people are often scared is some systems start and say, oh, you know, we're gonna, let's say, we're service company, we're gonna collect this data in that way, and then suddenly there is a backdoor, there's an integration with another service that starts to then violate the privacy and the original rule, and there's no room for recourse. And then suddenly you have this system, which are deeply integrated. It's actually one example of that. You may have heard about the Clearview AI system, what Clearview AI did was basically decrypt, all online photos, I mean, all social media photos from Facebook, Twitter, they made an app where you can take the picture of someone and all of the online photos of that person, whether they're in a private or public domain, will surface, because what they did is actually violated the user terms of social media, and they have actually script that data, and they have billions and billions of photo where, like, I think there was like a journalist that investigated, and when that journalist went, the Clearview person took the app, took a picture of them, and all of their data was, I mean, a lot of the photo that they didn't even know were online, surfaced,
- That's scary.
- And, including pictures taken by other people, right? So in an ideal world, like you would want say, such actors, that like infringe on the user terms and privacy condition to be punitive, but then it opens a whole kind of form because it's like, oh, wow! Now that you can do it, like, you know, you have a lot of people that would try to get in touch with Clearview, replicate data, duplicate the data, can I end up on, like all sorts of things that are really hard to control, and so I think like, your work, like the public and officials able to regulate these technology and prevent either legally or illegally I mean, by legal route or technical routes, the abuse, but often it tends to be a reactionary. So I would still say like, I mean, the conversation has gotten better as far as the technology development and like it could enable a lot of like powerful use case, but then we cannot, we have to be intentional about how to regulate and penalize the harmful use case. Otherwise, I mean, one example was also deep fake, where you can just make a video of someone saying whatever, and so now I can take your face, I can make you say whatever, and the technology is powerful and it can be done, right? So how do you then either from a legal or technological standpoint, fight against that, because we're already in the era of fake news, and we don't need more videos of someone that sell, like I can take someone's voice, someone's face, and make them say whatever I want, and use that to create more and more misinformation. So in that case, like the deep fake technology is very helpful for say, acting and animation, so the movie industry has actually really loved it, because now they can make editings and acting a lot easier. However, the deep fake also come with these harm and they cannot necessarily be technology stopped, so unless people and platform regulates and ban those acts, I mean, those technology, then we're gonna end up in a world where you have a mixed bag. So I think like thoughtful regulation is needed.
- It's also, it's a bit, it's also complex to just determine the situation by a particular database, because a lot of the issues with privacy deal with application of data. So even if one company says, we've anonymized the data, the way that people can have their identity leaked is, if there is auxiliary information or data that kind of leads back to reveal their identity. So there was a famous case of the researchers who were able to correlate the tips and the taxi routes of celebrities based on paparazzi photos. So sometimes data's unintentionally leaked or de-anonymized based on it's aggregation. So it's not as easy as just saying, oh, well, if this company makes sure that we protect the privacy of individuals, then we're okay, because you can still advocate data from one company and another company, and that can lead to issues of privacy.
- Yeah. It sounds complicated to regulate, but that's some, some sort of regulation seems necessary. Yeah. So I wanted to ask you a little bit about through Black in AI, what type of support, you talked about this a little bit earlier in the interview, but what type of support are you trying to provide other people getting into this space, that maybe you didn't feel was available to you as you were getting started?
- Definitely support for, well, I'm in grad school right now, so definitely support in general for the path to grad school. Grad school itself is a grueling process, and we have an academic mentorship program right now that is, would take place in, well, we're reviewing applications right now, but hopefully next summer, and I wished that I had that sort of guidance when I was applying to grad school. But at the same time, I want to provide where I want to be part of a process that makes that better, because unfortunately within the system of academia, as it exists right now, and it's changing slowly but surely, but it tends to reward individuals who already benefit from the process of being on the right path, but the way that we solve the most complex problems is by having as many perspectives as possible, and having a diverse and inclusive environment, and part of that means that we have to change the way we think about sourcing applicants who might be a great fit for academia, not just in terms of research, but the future professors in industry and in academia. So that's something that I'm very passionate about, and I think that Black in AI does really well in terms of supporting and in terms of this community, and it's something that we're working to make better.
- Yeah. I can go in detail with like many aspects because it's quite a deep question. And there's been a lot of great development in the past three years on that. So that we can start with, I guess I can start with like a few personal anecdotes. So when, I went to undergrad at MIT and perform research there, but I mean, often you would tend to be, definitely in your lab, you'll probably be the only black person, for sure. Maybe often it goes to your department. In my case, wasn't in a department, but I was the only person in my lab, and so it can always feel like, you can imagine being like at a dinner conversation and you're like there, but you're not sure if your presence matter as much. It's like you're there, but like, you could argue if you're not there, like the thing would still be the same. So you often question, you know, should I be in this field? Should I not be? Are there other things, are the other fields which are more appealing? And things like that. And in the case of like Black in AI, it shifted my perspective from you're not just solving, because usually if you stay in one field, like you would maybe do it because you like the process of solving the technical problems. So usually like people would say, I like this field because I like to do math, or I like to work on these kind of applications, but often if you're working on a really long-term subject, especially in research, it could be like five, 10 years away, the motivation can be, and I could encounter challenge, it could really affect you. But one of the big shifts was that, now it became almost like social pressure, because you're there, you have a community, people know you, part of your identity relies on that. So from that point, it goes from, I'm at the dinner table, whether or not I'm there, you know, it's like, whatever, but now it's like, this really engaging conversation in this house and like, even, it's like, oh man, it's like, I have to leave, but like, I want to leave, wait a second, no I can't. Like it's so great. And I knew people coming and they want to benefit from my experience from my perspective. So by doing that, it already works on the issue of attrition, right? Where like, it creates an environment where people feel, I really belong here, I can make a voice, I can make my voice heard. And there are role models, which are also accessible, and those people in power to actually pay attention to what I have to say, they help me and they can even unlock a lot of opportunities. So that's kind of, one of the starting point is that, because there was a really like high social nature to science, and so if you feel like your collaborators are really your friends, it makes a big difference because you're able to more freely suggest ideas and more freely question ideas. And so, I mean, as an anecdote actually, in my membership in Black in AI, I've been able to in the last three year, work on five papers, four are peer reviewed, one is currently in review, and that has actually happened out of school. That would not have happened without this community, because you just don't hear, because I worked in industry, people in industry who work on academic papers like that, that just would not have happened if I didn't have a network of people, I would go to the conference, there's a really interesting conversation, we have common interests, then we take it through online collaboration. So that's just like one concrete example. The second one is visibility of the work. So through the workshop, we enable the work that people do to have visibility and actually the best workshop submissions, they have a place like this podcast called "This Week in Machine Learning and AI," so the best work out of the community has visibility in terms of workshop. On our social media, we have around 20, 24,000 followers on Twitter, including really leading academics, like head of AI at Google, Stanford, MIT professor, people that like you, media, like everything. So it means that even if you're a person on Twitter with like 200, 300 followers and that you're on our radar, if you submit your work, we will retweet and get, you will get impression from 23,000 people. So that visibility and what's important there because as I told you, let's take the example of a person that not in like a university department with a lot of press, that person, when they will do their work, will be able to retweet that to a really big audience of people and give visibility to that work. And so that's like a third important one. And so when you start having this feedback cycle of I know that my work now can get visibility, it really change the mindsets. And in addition, actually, due to the success that we've had, Black in AI has garnered a lot of support and funding, and they're like even more ambitious programs that we're looking into. Some of the things in the work include supporting entrepreneurs from the communities, because for example, a lot of investors have approached us and say, hey, you know, we've seen amazing work coming out of the Black in AI community and we are interested in figuring out how we can now make introductions between people from your community and our firm. So it means again, that visibility and those barriers to access to these powerful people can be worked on, and now we have a partnership with multiple firms, then we could say, yeah, you should talk to that person. So those four things over time, they really make, they really contribute to like powerful presence and they all reinforce each other. And those are all of the four things which have been achieved over the last few years. And assuming that the momentum continues, really talking about unlocking all of these potential and changing, and it's like we really constructing this environment in a way that fits the community. And so it's like you're not just now trying to reform like any institution or advocate within any institution, you're really creating almost like an alternative environment that's more that's by design say, okay, how do we support a young person in that country? Because we actually have members from 40 countries, not just the US, like literally 40 countries, how to support someone that's in Brazil, they have taken three Coursera machine learning class, they need a collaborator, they need maybe a little bit of like, of grant money to work on a project with someone from the community. Like, that's the kind of design question that we have, and because we know the profiles, we can really say, okay, this is someone that would have typically been off the radar for many of these institutions, but we don't even just advocate within the institution we say, like we know you, and we're going to create a program that targets people like you, and those things are really game-changing.
- That's very cool. I saw online that Black in AI has uncovered some racist algorithms and things like that. Is that work that some of your members have done to sort of analyze and discover some of these things, or has that been mostly done by your founder? Could you talk a little bit about some of the findings that Black in AI has had?
- So there's definitely been work throughout the organization, not just, but I think that part of the advocacy came out of the founders and it has continued, or basically people have kind of been inspired by the work of persons like Luimi, Bebe, Tamika Brew, and so we've seen a lot of research. For example, we have the upcoming Black in AI at NeurIPS workshop where persons have done work highlighting things like inequity in health care. So we've seen during COVID that there's unfortunately inequity in terms of the amount of care that certain communities have obtained versus others, and so those kinds of workshops kind of bring a forum for persons within those communities to talk about this work and talk about the research that they've done. So we've had research in that perspective, and generally a computer version as well, that's another one. And another one that's people are interested in is FATE, which is Fairness, Accountability, Transparency, and Ethics. So we've seen things like text generation or issues of bias in texts, or even impact or research being done in African countries, and the different languages that are used. Because it's not the field itself is not very representative right now, we're seeing a dearth of attention being paid to non-Euro centric languages. And so having more black researchers by definition, is bringing more research in the forefront of low, or basically issues that primary academics in westernized countries may not consider.
- Yeah. And actually to add to that, I think in that case, I mean, one of the best way to look at it is that this section of AI, I mean, it's not the only work that the community does, but we well-placed, because we often live, from our live experience, that's where, like what a lot of the lived experience of the members suggest or nudge them towards. I think it's actually best to think of it as a field really, because what is a field? It's like a field is a bond of people will say these questions are worthy of asking, we're gonna work on them and build on top of each others work. And so seeing from that lens, like I would say a lot of the Black in AI members have actually led this subfield of AI and built on top of each other's work, and it goes from someone finding something as an anecdote to allow, this happens here, this happens here too, this happens here too, and suddenly you have a bunch of people building on each other's work and like mentoring other people to also ask this question. And so now, like, you'd say, I mean, I don't have a name exactly for the field, but like this field that center around the question of power and representation in the data set and their use case, it's becoming a subfield of AI that Black in AI members are really driving in, and the other one, the anecdote actually, on the African languages, so there's this paper called the Masonic Paper, that call about a participatory approach. These paper has 50 authors from the African continent. It was accepted at like one of the top NLP conference earlier this year, but actually as a result of that work, there's been an organization called the Lacuna fund, that has decided to give out grants to promote the creation of data sets on non-English languages. Because that is something that's needed. If we talk about AI, cannot just talk English, although most of the internet data is in English, but we also have to think about that. And so it went from, you could imagine one person being in one country, to say, there's no data about this specific language, to 50 people finding each other, doing work that gets publicized in a conference organization, like the Rockefeller foundation, the Lacuna fund, they see that and say, hey, this is actually an area that fits one of the goals that we want. We're gonna make a call for proposal for that to happen, and now suddenly you have these 50 people that have led this out that say, now you can be supported and create this field. And that part is really important because it creates agency, and it really brings the value of having other people, because it's not just other question. I mean, it's not just that they're gonna change, what if they're gonna really change type of question which are asked, and like that part is important because in term of, that anecdote it's really descriptive of like the value of different lived experience, and how the visibility of all of this work gets promoted, and there a kind of feedback reaction with other institutions, which are also thinking about how to empower members of other groups. And I think like one of the questions that could be asked is like, why is that needed? I mean, if people come from countries where there's a high fraction of the GDP that's allocated to research, like it creates these models, these other more global models of funding and doing research, really enable to take those lived experience as well, and infuse them in the design of AI, because if we really want to create this technology that enable all of the world to do XYZ, it's important to think about those lived experiences as well. So that just like a more in-depth example of how in that case, those sub-fields are created and propelled, but they would not have otherwise happened, if people hadn't found themselves and the capital wasn't there, and so it's one of like agency as well.
- A question I just would, I'll direct to you, Crystal, is it sounds like with Black in AI, you're able to really take someone who's already taken some initiative and getting into the field and like really propel them, but you both have talked about this issue too, of just like a lack of feeling of belonging, like is this for me because of the lack of representation, do you also have some initiatives to just try to broaden, like make people feel more welcomed into the field of AI?
- Yes, absolutely. So I go to a social on Tuesdays, at the interest of, it's the person who's in charge is a professor at the university of Michigan, Dr. Chad Jenkins. But one of the things that he says is that the biggest hurdle that black students face in grad school is isolation, and so a lot of the struggle and difficulty and feeling of not belonging comes from that feeling of isolation. And so Black in AI has by the different things that they've done, has created this space where people can meet each other, and there's kind of an act of self care that happens when you find other people like you, and they remind you that you do belong, and you find those spaces. And so we have created through Black in AI, opportunities for persons to not just find these spaces and interact with other persons like themselves, but also to bring that feeling of belonging and lead their own initiatives and communities within the spaces that they work and live. And so we've done some of these things by having reading groups, for example. We had one that was on causal inference, and we're having one on deep learning apparently, in early 2020. Another thing that we've had for, was it for ACL or ICML? It's just another--
- I think there was a social at ICML. Yeah. I think that was ICML. Yeah.
- Well, we had, it was really cool cause we discussed the decolonial AI paper, which was a massive hit this year, produced by two of our Black in AI members, and it was, which came out on deep mind, but it was also, so we discussed decolonial AI and what it means to us in a setting that included about 50 of our members. And we also played music, some people had poetry, some people spoke about artwork, and so part of it is not just creating a community to thrive, but engaging in self care, because that is, I think an incredible part of engaging in that pipeline, that leads to a long career with it for black students and black faculty, and black industry practitioners in AI.
- Yeah. That sounds pretty, that sounds pretty amazing. It sounds like from what you the two of you have said, it's done a lot for you individually as well. And lastly, I just wanted to ask if, how listeners to this podcast can support the work that you guys are doing at Black in AI.
- So yeah, there's a, depending on the level of involvement, out of the minimum is you can follow the work on social media, so on Twitter we are @black_in_ai, on Facebook, you can find the page. If you want to go a step ahead, you can find our website, blackinai.org and donate, and then if you are in a position of power in a university, corporation, that you can make a good case of why you can be a member of the community on the website, you can also apply to join, and we've seen, you know, we've seen all sorts of people apply to be recruiters that want to promote opportunities, faculty members that want to also promote opportunities to entrepreneurs that want to support the work that the community does, to investors that want to diversify their portfolio. So all of those things, depending on the level of involvement, there's room, so to repeat, you can follow us on social media, you can donate, and you can also join the community as an ally, if you are in a position to have on any of those points.
- Great. Thank you. And do you have anything that either of you would like to add before we wrap up the interview? Maybe like favorite COVID hobby?
- I took improv class last night, that is actually based in San Francisco, but, and I've also been engaged in a lot of reading groups, so I think that it's important during this time to remember that there are a lot of local bookstores that are really suffering on businesses in general, local businesses, and so I joined a reading group as well that's talking about unsettler colonies or just different topics. And so it's one way that I've been able to find community during COVID, supporting local reading groups and doing things like community theater, even though it's digitally. So I think that keeping safe and helping, support communities locally is really important, and that's part of why I'm engaged in Black in AI, cause I see it as part of my local and broader community.
- One other thing I do is a striving to walk around 30 minutes a day, like every day of the week cause I think like for the first few months, it was around, like be safe, be safe, but like you have to also look into how you are being safe home by like saying, am I like, I don't want to have other underlying health issues if I'm home all the time. So trying to get a bit more exercise, and striving for like 30 minutes of walk a day is something that I've been doing. And it's kind of nice, because like it's like definitely, I mean I'm based in Boston right now, there are definitely places of the city that I did not seen before. So yeah.
- Yeah. Physical and mental health is important too. I'm very impressed with you doing an improv class. Improv to me sounds like it's like one of my worst fears, I think just being put on the spot that way, but I'm sure it would be fun once you get over that. But yeah, thank you both so much for joining me. It was a pleasure to talk to you, and I think the work you're doing is so important. I think artificial intelligence is also really fascinating and fun to hear about some of the positive things that can come out of it, also very important for people to be aware of biases within AI and so that we can move forward hopefully in a way that accounts for that. So thank you both so much and we'll see all of you listeners in two weeks and thank you for joining us today.
- Thank you so much.
- Thank you.
- Thank you everyone for tuning into this special episode of the "iPhone Life" podcast. We'll see you again in two weeks and thanks for listening.
- Thanks everyone. We hope you enjoyed it.